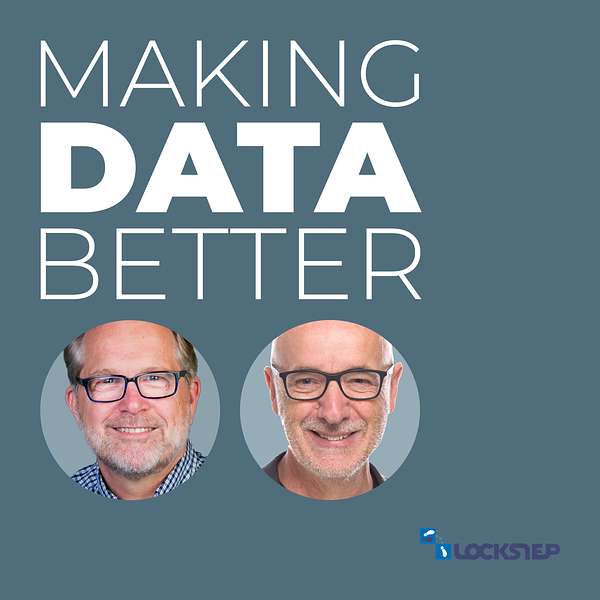
Making Data Better
Making Data Better is a podcast about data quality and the impact it has on how we protect, manage, and use the digital data critical to our lives. Through conversation and examination, George Peabody and Stephen Wilson look at data's role in risk management, at use cases like identification, lending, age verification, healthcare, and more personal concerns. Privacy and data ownership are topics, as are our data failures.
Making Data Better
EP16: Data, Governance, and Public Service: Ian Oppermann
The focus of computer technology historically has been on the manipulation and communication of data and information. Yes, there’s always been the monstrously obvious admonition of “garbage in, garbage out” when speaking of data. But as our dependence on data grows, the issues of data quality, of making data better, have grown in importance and complexity. Data, it turns out, is endlessly nuanced.
Government Data Generation and Usage
Government has an enormous interest in data. It is an issuer of data when it assigns account numbers, for example, to its citizens to ease service delivery. It is also a considerable consumer of data in order to establish policy, measure program efficiency, support planning, and, just as with any business or individual, for decision making of all kinds.
But this isn’t simple. The term “government” masks the fact that multiple agencies exist, each with its own goals, never mind data handling policies and procedures. Sharing data across industries is as nuanced as data sharing between enterprises or even more so.
Understanding how governments think about the data they consume and generate is key to long term data security and online identity.
Talking with Data Expert Ian Oppermann
In this fascinating and stimulating conversation, Steve and George discuss these topics with Ian Oppermann, the former data director for the state of New South Wales, a director for Standards Australia, and advisor to multiple startups.
Ian shares his insider’s knowledge of government agency priorities and the fact that sharing data across agencies is “extraordinarily hard.”
Just at the Beginning
Standards Really Really Matter
Ian’s participation in ISO standards development comes from his insight that data sharing requires very crisp definitions, detailed use cases, and specific guidance for each use case based on privacy and data custodianship requirements. And he points out that we are just at the beginning.
For example, the latest ISO standards tackle the basics of terminology definition and use cases, ISO 5207, and guidance of data usage, ISO 5212.
These standards do address the use case of AI but even at this stage the standards address the basics.
People Matter
As with many technology management concerns these days, the concerns are rarely about the tech itself. They’re about people, too. Here’s Ian:
“If you want to use [data] for important purposes, you actually need people who know and understand what data is, who know and understand what data governance is, and who know and understand how to actually use the data for appropriate purposes and then put guidance restrictions or prohibitions around the data products you create.”
Ian concludes with:
“But [for] the general use of data, we're only just beginning to understand the power, the complexity, the mercurial nature of data and starting to build frameworks around it.”
Take a listen if you care about data management and governance in large organizations. We are just at the beginning of getting this right.
Welcome to Making Data Better, a podcast about data quality and the impact it has on how we protect, manage and use the digital data critical to our lives. I'm George Higley, partner at Blackstuff Consulting, and I'm delighted to join my partner, steve Wilson. Great to see you again. Let's get right into it. We've got a terrific guest today, ian Opperman. Among his many accomplishments and we'll ask Ian to you have to brag about them yourself he's on Wealth to Check a couple of years he's advisor to a legal tech startup. He was struck by your role as a professor at the University of Technology in Sydney, most recently as chief data scientist for the state of New South Wales. Nsw has been a leader in data analytics and open data. So, ian, we're really delighted to have you here and look forward to hearing your thinking and a bit of the story of New South Wales and the Digital Driver's License and a lot of other topics, all right. So, Ian to you, love to hear about what you're doing today.
Speaker 2:George, great to be here and great to be talking about data quality. As I mentioned during the introduction, I was the Chief Data Scientist for New South Wales government for eight and a half years, an experimental role which was really about helping government think about data differently and see data differently. Now left and I've joined a startup. Of course, the thing you do after being in government is join a startup and that startup is all about helping governments do government differently and, of course, using data, ai and digital tools to do things differently. Joined with a former minister, the former CEO of what's called Service NSW and the former minister's chief of staff, and we're out there doing good things with data, digital and AI to help other governments around the world.
Speaker 3:Fantastic, and I myself had the privilege of consulting to Service NSW on and off, especially through COVID, and seeing firsthand you know the agility of that organisation. It recently won an award actually for service innovation, which was just such a great result and a real testimony to the quality of the people there. The innovation included things like the Data Analytics Centre which Vian I think you had a title role of some sort there, but maybe you could tell our listeners about the importance of data and how New South Wales really sort of walked the talk in open data and open government.
Speaker 2:So I joined New South Wales government in 2015 as the first ever chief data scientist and was given not only the gun and the badge, but also a piece of legislation that said you had to share data with what's called the Data Analytics Centre, and the centre was all about tackling wicked policy challenges or problems that are complex, subtle and ultimately have people's behaviour at heart. We adopted the philosophy that if we're going to tackle a wicked problem, we need to deliberately re-complicate that problem and try to address as much of it as possible, and that, I have to tell you, was really an unusual philosophy to take in the government, which typically is about keeping it simple. That was helping government think differently about how to use data to understand problems differently, use data sets a myriad of different data sets to see the problem differently. Every data set is incomplete. Every data set is less than 100% quality. Every data set doesn't give you full coverage, but it's a point of life, and the whole philosophy was let's see how many different ways we can understand that problem and then use analytics and AI to really make sense of it and do things differently.
Speaker 2:The flow I was taking to Service NSW was now we better understand the problem. Let's deliver differently, and my colleague, david Rees, was really the champion of Service NSW. He took the philosophy that government can, government can do this, government can be agile, government can experiment, government can try, test and learn and build out services in an agile way. We had all been working together for some years when COVID hit. And when COVID hit, we were ready to respond and that was the really big difference between before and after that beginning of that experimentation in 2015. When COVID hit, we could respond with data-driven tools and data-driven elements of identity and data-driven ways of delivering services, because we'd spent years experimenting up to that point.
Speaker 3:And of course there's a really good social contract, I think in Australia at least, for the use of data, the reasonable use of data. You know our COVID response in New South Wales is very strong through New South Wales Health. The public more or less embraced the idea that contact tracing was going to be a thing and you know we had such a rash of private sector contact tracing cloud solutions. It was a mess and I think it was Victor Dominello himself who said we should make this easy and uniform for citizens.
Speaker 1:But I also want to like tag.
Speaker 3:The point you just made about complicating things, I think is genius. I'm a mathematician and scientist by training and I think about generalizing and in engineering we try to simplify, look for simplifying assumptions. In science, sometimes we're looking for that grand general theory. Usually generalization makes things more complicated, but in our area of digital identity, we think that generalising the digital identity problem to a data problem surprisingly makes things simpler. That convergence I'm going to come back to that later, the convergence of governance of data and ID. But you know, for now can we go big and talk about data sharing, because I think that that's one of the defining themes of the digital economy. We're doing open banking in Australia. We've got open data. How do you think about data sharing generally and what are the societal parameters that you think about?
Speaker 2:So, steve, you've addressed the entire Pandora's box with your set of comments there. Let me try and unpack the sub-elements of it. The first was around social licence, and it's an interesting position to be in government and be talking about social licence. Social licence is typically associated with mining companies and such things. You don't get a choice about engaging with government. When I started in 2015, there was this real concern that if government was doing data analytics, if government was doing analysis of data at a sort of at scale, that there would be this concern from the public and the perception of public perception really inhibited a whole lot of activities. It wasn't the public saying no, we don't want you to do this, it was inside government people's thoughts about what the public might say. That was really a limiting factor. So we went out there and tested it. We got the data and the data was, quite simply, we don't want to answer the same question because we want to deal with one government, we don't want to deal with 15 different parts of government. So we actually spent some time using the data to understand how people felt about government using the data Absolute genius. We also had the leadership you mentioned, victor W Lowe. We had a minister who said this is ridiculous. Government cannot be this complicated to work with. It has to be simple. It has to be like dealing with service companies, because government largely is a service. So we had the most high-level leadership constantly battling the ridiculousness of engaging with government, which gave us all a license to do it better and do it differently, to reimagine how government can actually work. When COVID hit, the ministers said we are going to do things differently, we are going to do things simpler, we are going to make it easier to engage Into. The line here, of course, is we want people to be able to live their lives within the constraints of COVID and what people are likely to do so simple things like QR code check-ins and QR code check-outs, simple things like delivery of vouchers for people to try and stimulate the economy was all done because we all felt that we were enabled and allowed to do things differently. And, of course, service New South Wales was the vehicle that allowed that delivery. Data Analytics Center was the place that allowed us to understand where best to place those resources.
Speaker 2:But all of that requires data sharing. So, steve, I'm catching up with your series of questions. All of that relies on data sharing and data sharing, especially within Google, is extraordinarily hard. There are three broad sets of reasons why people aren't sharing data. They're unwilling, they have concerns about all sorts of things, they're unable, they don't have the frameworks or they believe they're not allowed or actually not allowed to do data sharing.
Speaker 2:And two of those changed really dramatically during COVID. People were willing to share data. They really understood by that stage the importance of doing it. They also had legislation that said explicitly you will and you must share data in order to make things better. And so, if we got down to the frameworks of the unable, how do you share data with appropriate governance, especially data about people? So privacy, especially data which is sensitive, so sensitive subjects and particular health is a sensitive subject. Building out those data sharing frameworks that we had been experimenting with for years suddenly became important to put into action. From an advancement of the maturity and data sharing and use within government, covid was amazing. It was, of course, the turn of the maturity and voucher use within government, covid was amazing, but it was, of course, a terrible pandemic. But from the mechanism to move us forward, it was amazing from the perspective of everyone's maturity and focus improved for the volume of voucher.
Speaker 3:Yeah, absolutely Necessity, classically being the mother of invention in that case.
Speaker 2:So we had been inventing for years, but it was all kind of interesting experimentation. The difference was we had to deploy it. We had to actually stop experimenting and actually do so. In this case, necessity was the mother of implementation.
Speaker 3:Nice one. I like that twist. Look, there's other things going on, a lot going on. We've got a Data Availability and Transparency Act at the federal level in Australia. We've got national data archives. We've got things like the fear principles that are supposed to provide a governance for data sharing that are supposed to provide a governance for data sharing. We had that sort of metaphor about trying to describe data as an asset or as a resource. But how do you on the economic importance and maximize the economic outcome of data as a resource?
Speaker 2:You see you're touching on some very, very big issues. Let me try and approach this from a couple of different perspectives.
Speaker 2:You talked about the Data Evaluating Transparency Act. Legislation describes things at the principle level and that's great. That says this is how we're going to do this, but it's a bit like describing the principles of changing a tire or a bit like the principles of how you're going to wire up your house. It's useful in the sense of saying this is what we should be doing, but it's not practical in the sense that you consider a group of people and consider them talking about changing a tyre on their car at a principles level. But someone needs to pick up the tools. Someone actually needs to get in there and do the doing, and a lot of the work so far has all done. Whether it's the Intergovernmental Agreement on Banacharing versus the Banacharing-Rose Act doesn't help unless someone is willing to pick up the tools and a lot of the good intentions. During COVID we had the Intergovernmental Agreement on Banacharing that says you are allowed, we are willing and you're allowed. So out of the unwilling, unable, allowed, it spoke to the two ends of it. It didn't help with the frameworks and starting to consider data as actually something which is not just an Excel spreadsheet, not just something that you do a little bit of analysis on If you want to use it for important purposes. You actually need people who know and understand what data is, who know and understand what data governance is, and who know and understand how to actually use the data for appropriate purposes and then put guidance, restrictions or prohibitions around the data products you create. That thinking still doesn't largely exist in the world, so I don't agree.
Speaker 2:There are purposes. People use data for a very, very narrow, specific purpose or they use data in a very narrow, specific context. But the general use of data. We're only just beginning to understand the power, the complexity, the material nature of data and starting to build frameworks around it. Most people think of data as an Excel spreadsheet. That's the mental model most people have. The practical reality is if that data is important or to be used for an important purpose. It's really important to understand where the data came from, how it got to, what the chain of custody was, what the authorizing frameworks are, the elements of data quality, and then assess whether that data is fit for the purpose you want to use it for. And then when you create something from that data whether it's a summary or a subset, or an alert, an alarm, a decision or an action or even just an insight. It's really important to understand how to contextualize appropriate use of that data product and put guidance, restrictions or prohibitions on how you use that insight so that it can appropriately be applied for important purposes. If you're using data for fun, it doesn't matter. If you're doing correlations between by consumption and political outcomes, it doesn't matter because that's a fun pilot trick. It matters when it matters. And if it really matters, it's important to understand the data before you use it, as you use it and after you create those data products.
Speaker 2:That understanding is opening just a door to a lot of people, I mean for these analogies of the past. You know diaries of the new world and that's such a terrible, terrible analogy. It doesn't fit in so many different ways. I guess the assumption is that it's plentiful and you can create value from it. But that's completely wrong.
Speaker 2:Data is much more like the new electricity. You have to really evoke yourself if you apply data inappropriately. And we all, of course, know that. You know we all have that basic understanding of electricity from a very early age. We know not to stick a fork into a toaster. We know not to stick our tongue into a light socket. But each and every one of us can make a toaster. Each and every one of us can change a light bulb, but if we go to rewire a house, you know you need to get an expert in electrician. If you're going to build a power station, you know you need a group of really experts to do that.
Speaker 2:But with data, we all think that we're the home handyman. We just pick up the power tools and drill holes in walls and do silly things with data. We need to get to the point where we understand that data is extraordinarily powerful or can be extraordinarily powerful, and when it's extraordinarily powerful, we need to build the right frameworks around it to ensure that we do it in a way which is appropriate and we put restrictions, guidance around the data products we create. The alternative is what happens now, at least within government People won't share data because they're concerned about what you're going to do with it and not only you, but the next person and the next, next person will do with the data or the data products or they're concerned that someone can stand on one leg and squeak and re-identify an individual. So we either don't share or we boil the goodness out of it and release open data, which is not that useful and really really open data sets are just not that useful.
Speaker 2:You asked a very big question. You made a very big statement. Data sharing can be done in systematic ways those people sitting around looking at the tire and using principles to try and get that tire changed. Someone needs to pick up the framework, someone needs to pick up the tools and do the doing around data sharing frameworks that actually make sense. But consider, before you use the data, the fitness for the purpose as you use the data, and what to do with those data products once you create them.
Speaker 3:So you said a number of things that are just fascinating. You're touching on research, and I think it's a word that is underestimated. I think that the word has been bastardized by the slogan do your own research, as if the person in the street can analyze data and understand it and reach robust decisions. I think that we've cheapened the respects institutionally for professional research, but there's a fork in the road here and we could go both ways, and you've been involved with both of these. One of them is the economics of data, or the beautiful book Infonomics that you pointed me towards, ian, a couple of years ago, so we'll put this in the show notes.
Speaker 3:But Infonomics, I think, was a at work about 2018 and I think it was focused on almost like a taboo topic, which is how do you, how do you realise value, how do you monetise data, now that there's a lot of dark surveillance, capitalism and a lot of dark agendas behind that idea. But I think that Laney was trying to make that idea maybe, but I think that Laney was trying to make that idea maybe respectable or governable. So that's one thing, and we could talk about the economic outcomes whether or not using economics to get social outcomes is a good idea, and the other one is data quality. You drew attention recently to the new ISO 8000 international standard on data quality, and I guess that's going from principles to tools, isn't it? So can you walk us through whether or not data quality as a standard is something that's going to lead to some good outcomes as well?
Speaker 2:Once again, you've struggled me until I had to do a box. Let me see if I can step through some of those, but that lady's got infinite knowledge.
Speaker 3:It's a treasure chest, isn't it? It's not all problems and poisonous snakes.
Speaker 2:It's a chest of some sort with some stuff inside it, and what you make of it is whether it's treasure or Andorra's box. So that lady tried to build a framework that said data is valuable. We all think about that analogy data is the new oil. As much as I hate it, the intention there is to try and say it's valuable, but no one's really sure how it's valuable. So what we've done tried to do was put a framework in place that said you can use data for operational purposes. You can use data for strategic purposes. You can use data to create insights and all of that's sort of the internal uses of data. And there are more than simple operational purposes. You could and should be using your data for strategic purposes.
Speaker 2:Most organizations do not. Most organizations don't realize the value of their own data. They also center around some external value aspects to data. What would someone claim for it? Or what markets would you create? Or what new value would you create if you mixed your data with someone else's data? And then, of course, there are elements of putting up potential loss as well. What would it cost to reproduce the data? What would be the impact of you not having exclusive access to that data and I thought that was really, really good. And then what I'd been trying to do at the time was work out a government version of that. So he talked very business-focused. I thought what would government do with data? And I'd also been trying to work out how we can build frameworks, literally an accounting framework for data. So it was a very nice meeting of minds around that idea. We are, to this day, still without an accounting standard which values data and tell.
Speaker 2:Facebook that their data is not valuable. It is the majority of the value of the company. Tell LinkedIn their data is not valuable and it's the majority of the value of the company, but we don't have a way of measuring it from a finance perspective. So that problem is still being tackled. But I think we're getting better at saying it's in here somewhere. The problem's in here somewhere. We don't quite know what it is, but it's in here somewhere. The problem's in here somewhere. We don't quite know what it is, but it's in here somewhere.
Speaker 2:Data quality is a really interesting element and as much as I hate the oil analogy for data, I often try to help people conceptualize by saying that data comes in different qualities and you can still use poor quality data, but what you can do in terms of reliance on the outcome is quite different. So it's like putting in Australia we have 91 grade, 95 grade, 98 grade petrol and we have diesel and you need to know whether your car needs to have or can run on 91, 95, 98, or whether it runs on diesel. You need to make sure you absolutely don't mix them up, but you can still run your car, your high-performance car. It can still run on 91. It just doesn't work so well. Or you can run your clapped-out car on 98. It might be good things for the engine. The point is you need to understand if it's fit for the purpose you want to use it for. We trust when we go to a petrol station, we trust that all the work around, where it came from, how it got there, that chain of custody, that chain of problems we trust all that away because we trust the brand of petrol station. But we still have to make the assessment for ourselves. Is it fit for the purpose I want to use it for? Would I inadvertently put diesel into my petrol engine? Would I inadvertently put 91 into my high-performance engine, which requires 98? So we still have to make that decision.
Speaker 2:Data policy is, if you think about all the effort, all the engineering, all of the process, control, all the governance that goes into getting that petrol into the bowser with data, we just say I've got an Excel spreadsheet, let's just pop it in and see whether we get some insights out. We need to put the same effort into the data in order to get the same reliance on the data products that we create. And again, those data products can be really, really broad. It could be a plot, it could be an insight, it could be an alert, an alarm, a decision for action. It could be any one of those things, but in order to rely on that, it's really important that we've done that work around the gardens.
Speaker 2:There are several things that I looked at in the past as chief data scientist, where I ran what's called the AI review committee, and AI is a use of data. We looked at really important uses of data like sepsis prediction, emergency department patient emergency department is about to go through a sepsis episode. An algorithm says alert and someone rushes over and treats the patient. That is literally a life and death use of data. The alert is not a decision. That arc of alert alert decision action. It's not doing something, but it raises the attention of a human being who responds in a heightened state of awareness to say this patient is about to go through a sepsis episode. Getting that wrong either false positive or false negative can be really visibly impactful to that person. It could be literally life or death. In order to trust the data, in order to trust the data product, we really need trust in that governance process that got us to that point, because if someone's life depends on that alert. Even though it's a human alert, that alert leads to a heightened state of awareness around responding to a life-critical condition. We really need all that governance to be in place.
Speaker 2:So data quality is really important and the standard you enter the 8,000-series standards comes in many, many parts. It actually says to us that data quality is not the issue of whether you've got day-to-day, month-to-month year-to-year or month-to-month day-to-day year-to-year. It's not about simple things like that simple format of things. It's about the entire governance process of data. How does data flow into your organization? What are the controls and the chain of custody, the chain of authorizing frameworks? How did the data get collected?
Speaker 2:It speaks to if I hesitate to say the life cycle of data, because life cycles in data are either trivial or infinite.
Speaker 2:So it tries to speak to life cycle of data, because life cycles in data are either trivial or infinite. So it tries to speak to the element of life cycle and it says these are all the things you need to consider. Let me revisit that analogy of the petrol station. It allows us to re-imagine that from a perspective of data and say there's a lot more to this than just bank format. There's a lot more to it than whether you've got the year right to one decimal point or the number right to one decimal point. There's a lot more to it and it's really important to know and understand that. If your use of data is important, that you really have to at least be aware of these standards preferably have someone you've organised and you understand these standards or know where to go to get that extra help in order to enact these standards, to have appropriate confidence and therefore trust in the data before you use it, the data as you use it and the data after you, the data products you create, what you carry around those and the data.
Speaker 1:After the data products, you create what you can around those. Well, ian, steve and I, as we talk about data sharing, we're often using the term data supply chain, because you've got issuers of data and they often get the data to the party that needs it to make a decision, which should be multiple entities. In between touching that data, you talk about data provenance and recording the evidence of that supply chain journey. One of the major producers of critical data is government itself, creating Medicare numbers, driver's license numbers and many of those my birth records and the like. Many of those numbers are used very commonly in commercial contexts, whether that was my design or not, but they still are very important for use for onboarding purposes, for example, creating an account relationship.
Speaker 1:So, stephen, I've been thinking about government, as, of course, you're issuers of these numbers. Thus far, they've really been issued either on paper or in plain text, and plain text, of course, is the target of every breach and the whole planet is affected. The number of producers or it's all led to go any closer to identifying me as a safe driver or as a driver? Or why is this driving me to South Wales? Why don't we turn that into a verifiable credential that has security. It's presented out of a wallet, out of a security device, so that hardware and software will be together. That's the way we think it would be very helpful for government to expand its remit, if you will, in terms of that data issuer Really providing a more useful to society version of the data that it's already producing. How hard is that?
Speaker 3:How hard is it to get.
Speaker 1:Again. You're laughing. That tells me something already. How hard is it to get government to expand its thinking about? If we are issuers of data? How can we get that into society in a more useful way?
Speaker 2:So if I were still with government, you would just ask the career-limiting question. So thank you for asking that.
Speaker 1:Well, that's also delighted. You aren't in government any longer.
Speaker 2:Yeah, it's not that long ago, so I'm still a little shy with questions like that. It's really hard for a couple of reasons. Let me wind back. Let me try, and perhaps that question must have a stake. I'll be going, so I'm still. I'm sure I've written questions like that. It's really hard for a couple of reasons. Let me wind back. Let me try and approach that question much like I have with Steve. You've asked a really important and big question. The first part of that is, of course, that's what we need to do. We are stuck in the 20th century thinking about creating honeypot kind of sense, where we bring all the data together and make ourselves a target for cyber attack. Once upon a time you had to be interesting for someone to put the effort into trying to attack you from a cyber perspective.
Speaker 2:Nowadays we're all just collateral damage. You don't have to be at all special. Every data set is going to be attacked at some point. It's just the nature of the world we live in. So let's not create those data sets in the first place. The problem is most people in government can't see a different way forward. Most people wouldn't think that that's just the way you do stuff. You just build data sets together. So there is an important mindset change that must happen. That isn't yet happening. So that's the real problem. Virtualization of data is a way of doing this. Being able to give people you were hinting at it, let me make it explicit giving people their own explicit control of their most important data assets, such as their identity, is really an important way of thinking about how we should go forward.
Speaker 2:Most of the time, I don't need to ask you the question what's your date of birth? I'm asking you the question in front of government are you old enough to enter this license premises? Is A greater than B? And if the answer is yes, then off you go. I don't need to know your date of birth. I don't need to know where you were born. I don't need to know all the sort of stuff that people typically will take from you.
Speaker 2:If you try to enter a license for purposes, I don't need to know whether you have a responsible. I don't need to know your date of birth, whether you've got a responsible service of alcohol license. All I need to know is is it valid? Do you have it? Is it valid? Is it within date? And the answer to that question then allows that next step to happen. That thinking is a step beyond where most people are, because we're so used to having physical identity that we hand over, which has lots more information that is actually required to answer the question are you old enough to go on this license premises? But that's where the work needs to go. Sorry, george.
Speaker 1:So I totally get your answer. What is it that people like us need to do to change that mindset? I mean, honestly, that's kind of the mission of why we might even be doing this podcast. We want this discussion to be out there.
Speaker 2:Okay, many, many times I've sat around a table with government colleagues and listened to their plans for how they're going to do things in future and thought to myself you're kidding, you're absolutely kidding. Really, this is what we plan to do and then try to help people understand there is a way of doing things differently. The hardest thing in the world to do is change someone's mind If they're set on a path of action they've got their planning, they've got their processes, they've got their resources, they've got their budget and you try to say to someone don't do it that way, do it differently. It's really hard to do. You're an annoying barking dog on the side of the road where they're on a mission to do something. It genuinely takes a crisis. It genuinely takes a crisis to help people think that they can do things differently.
Speaker 2:Covid was an amazing agent for change for the way we think about data. Data breaches, unfortunately, are an amazing agent for change. When it impacts a group, they suddenly listen, they're suddenly ready to do things differently. But watching everybody else do things or getting good advice, that's. That's interesting, george, but I'm busy, busy doing grown-up stuff over here. I don't really need your advice. So, unfortunately, in some cases, you have to live through a couple of crises, unfortunately. You have to help people when when they fall in the hole. You have to help people when they fall in the hole. You have to help people out.
Speaker 2:Let's say this is allowed doing things differently and the really, at least in Australia, there's been a tendency for governments to de-skill over the last decades. So we have a lot of generalists in government who are not technical, who don't get the next level of detail and who just think well, you know, this is what we do, this is how we do it. So there isn't a simple answer, but being there to help this thing, these are elements of how we get going. So I hope this podcast, I hope someone listens to this podcast and says, oh, maybe I can do things differently, maybe I can look at this differently, but it's very hard to change the mind of someone. It's really hard to change the mind of a public servant. It's really, really hard to change the mind of a public servant who's got all their pieces, their program logic laid out and their budget and their resources are ready to go. It's really tough to do.
Speaker 3:We've got other catalysts that I guess are programs that governments have extensively committed to, like Digital ID now and open banking, do you find? Well, it's a leading question, ian, but we find patterns through these programs that are common and they suggest to us maybe there's an emerging sophistication about how to govern data, what the parameters are that are governable, what can be standardized, what should be decentralized and left to be determined on a local sort of basis. One of the anxieties I have about ISO 8000 is that we had to see this process or this movement become too robotic in the way that ISO 9000 did. It poisoned the well by making quality management like an algorithm or a spreadsheet and it took a lot of the judgment and intuition and humanity out of quality management. And you know 30 years and I think ISO 8000 is going to do a better job. But are you getting to the point where you can imagine how this weird, intangible thing is going to be governable in a general way?
Speaker 2:Thank you, steve. Once again you've answered the right question. On the record, I love standards. I'm a huge fan of standards. Without standards, we are electrocuting ourselves, we are sticking our tongue in the light, we're doing all that dumb stuff. There is a tendency for standards to get a little brilliantly focused, a little heavy, and you touched on an example right there. I think there are other standards that get so cumbersome that they become less useful than they could be, but here's one I prepared earlier.
Speaker 2:We've just finished, after multiple years of work at ISO IEC, so at JTC1 standard on data usage 2.5207, terminology and use cases. Believe it or not, defining the terminology, getting the definitions right, is something that largely didn't exist or happened in a multitude of different environments, and we fought like cats in a bag about getting those definitions. Because the cloud people think this, the document exchange people think this, the AI people think this, the smart cities people think this. We said let's get that down. And then guidance for usage, and one of those usages, of course, is AI. So we connected to the world of the AI folks, we connected to TAO, we connected to all this stuff and we said you know, it needs to be simpler. It also needs to acknowledge that there is uniqueness in every circumstance.
Speaker 2:There is some of that judgment that's required, but the formula for these standards is, quite simply what do we need to know about the data before we use it? What do we need to understand about the data as we assess it for fitness purpose? What are the guidance, restrictions or prohibitions we need to put around the data product? We've read the thing that I've been sort of slipping into the conversation to know is exactly what's in that standard, and I think it balances the slipping into the conversation to know is exactly what's in that standard, and it acknowledges the fact that context matters, your environment matters, your sensitivities matter, your particular circumstances about your data matter, but most of it 80% of it, by the way actually is a repeatable process. But the 10% is your judgment and your context and your environment and you need to do some level setting about layers of control very high control, high control, moderate control, low control, no control.
Speaker 2:You get to decide, but this is how you decide. This is a recipe for you to decide. You still make that dish, you still bake that cake. It's your cake, but this is how to turn the oven on. This is the temperature to turn the oven at. This is how long to think about it should go on the oven for. But you're still doing that subjective confinement to not make it robotic, because data is really complicated. It's so complicated that in fact, there are seven degrees of infinite complexity in data sharing and use. Seven degrees of infinite complexity, data sharing and use, which we're going into a lot of trouble. But that basically comes down to every data set can be used for an infinite number of purposes. They might not be fit for purpose, but you can use it for an infinite number of purposes. Context changes the sensitivity of data, so you bring that data set close to another data set or you know something about something in the data. The context of use changes it.
Speaker 2:All those ambiguities, all those possibilities, try to create the things you create. Whether it's a salary data set or a self-set or an insight, that same issue exists, that context matters, and you can use that insight for a range of different purposes and there's a couple more steps in there, but it means that it's so mercurial, it's so difficult, so slippery, it's like smoke, it's like trying to eat your arms around something which is really dangerous. So if you can flatten all those ambiguities or uncertainties into a couple of different types, layers of control, then you can start to do something useful with it. That's what these new standards talk about. It says this is what we mean, these are the definitions and we mean the same thing consistently and these are the ways of thinking about how you use data. And therefore it's 5.7,. Terminal Newscast is 5.212, a guidance for usage, and one of those usages just happens to be AI.
Speaker 3:Brilliant. Let's get those details from you and put them in the show notes later on and see how much of that material is accessible to our audience. It's fantastic guidance. Thanks, Ian.
Speaker 2:It was a lander of love. A lot of landers and a lot of issues. People who try to get their arms around it start to talk about things like what's the difference between data and information. That one's easier to dial out, but then information and knowledge and wisdom. It was three in the morning. We were really close to the end of the standard. Someone said we haven't talked about wisdom. I said no, the ISO definition of wisdom. We'll probably understand it. If not, we're ending this conversation. Wait, I love it.
Speaker 1:Well, I think it behooves us to exercise some wisdom at this point and to bring this to a conclusion. Ian, thank you very much. I'm really grateful for your insights and your thoughts. I'm going to be thinking about how we shift government minds when they already have all the momentum in the world for a particular vision and we're using them over slightly.
Speaker 3:Thank you very much. Thanks so much, ian. Here's to data and information and, if we can get, to wisdom. Thanks for helping us along the way Later. Thank you.